Decoding VAE: Its Role and Necessity in Stable Diffusion
Decoding VAE: Its Role and Necessity in Stable Diffusion
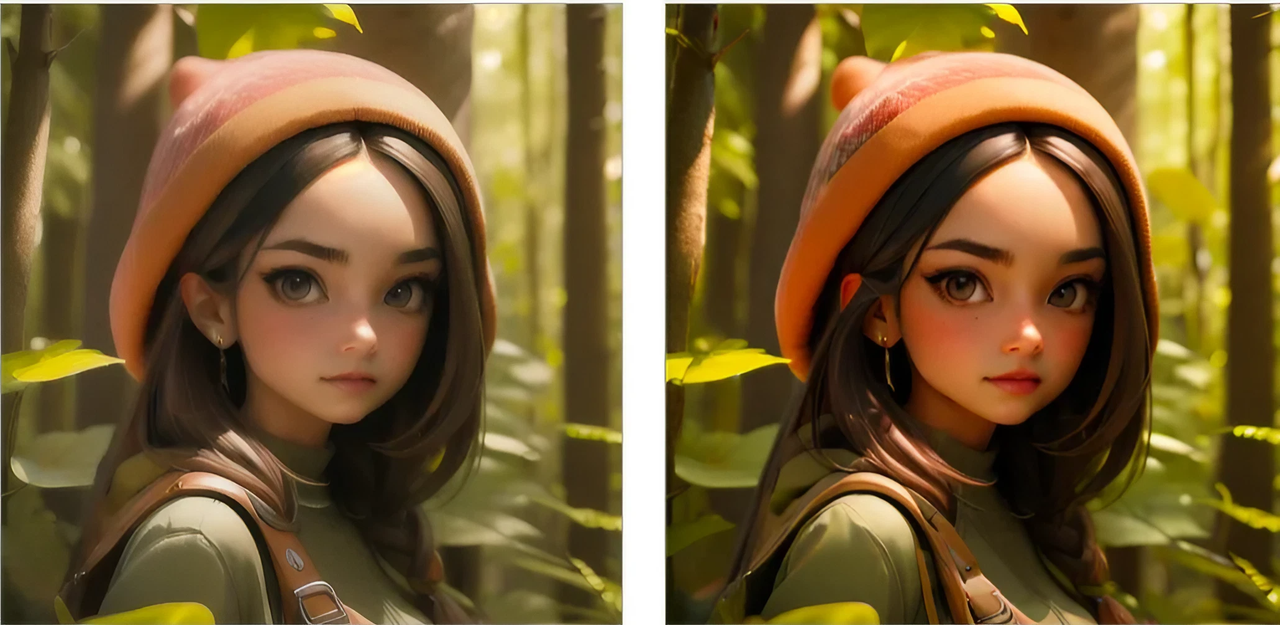
Introduction:
In the intricate world of data science, understanding various models and techniques is crucial for effective problem-solving. One such model is the Variational Autoencoder (VAE), which plays a significant role in Stable Diffusion. This article aims to shed light on what VAE is, when it's needed, and its necessity in Stable Diffusion. Whether you're a seasoned data scientist or a beginner in the field, this guide will equip you with the knowledge to understand the role of VAE in Stable Diffusion.
What is VAE?
The VAE operates by encoding an image into a latent space, a compressed representation with fewer dimensions compared to the original image. Subsequently, the latent space is decoded to generate a new image, typically of superior quality to the original. Within Stable Diffusion, there are two primary VAE variants: EMA (Exponential Moving Average) and MSE (Mean Squared Error). EMA is widely regarded as the superior choice for most applications, producing sharper and more realistic images. On the other hand, MSE can generate smoother and less noisy images, albeit potentially sacrificing some realism when compared to EMA-generated images.
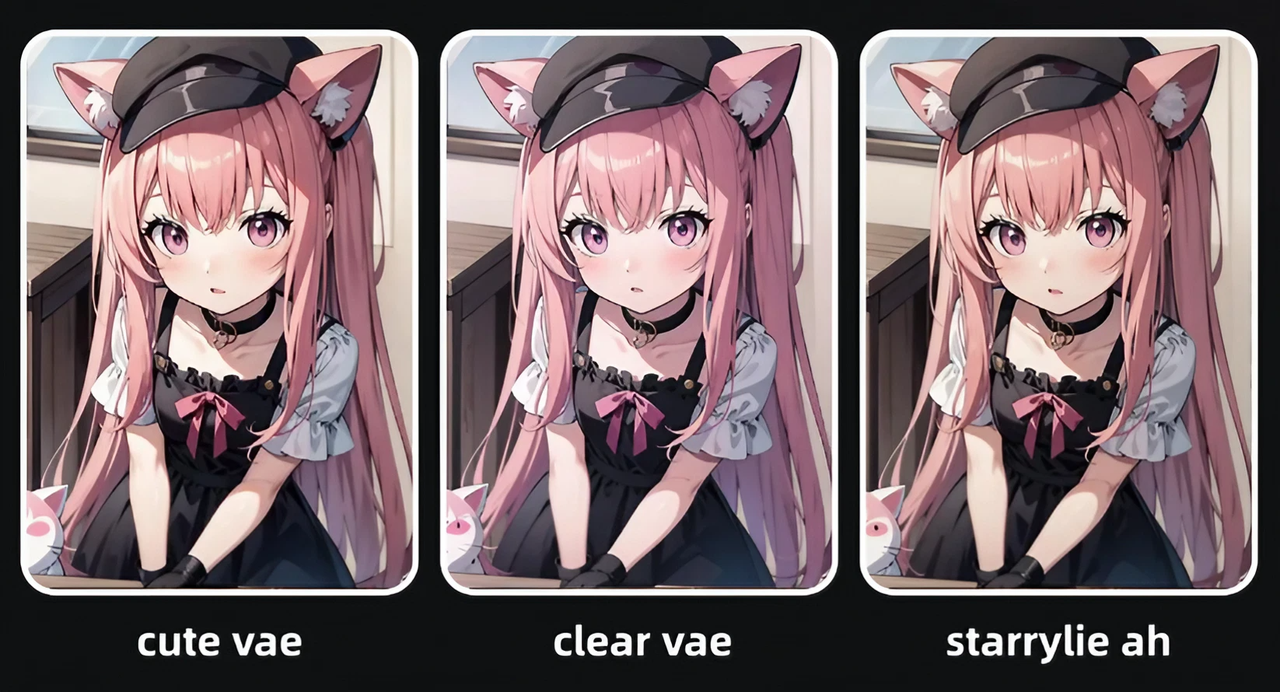
When is a VAE Needed?
A VAE is needed when you want to generate new data that is similar to your training data. This is particularly useful in tasks such as image generation, where you might want to generate new images that are similar to the ones in your training set. VAEs are also useful in tasks that involve dimensionality reduction, anomaly detection, and more.
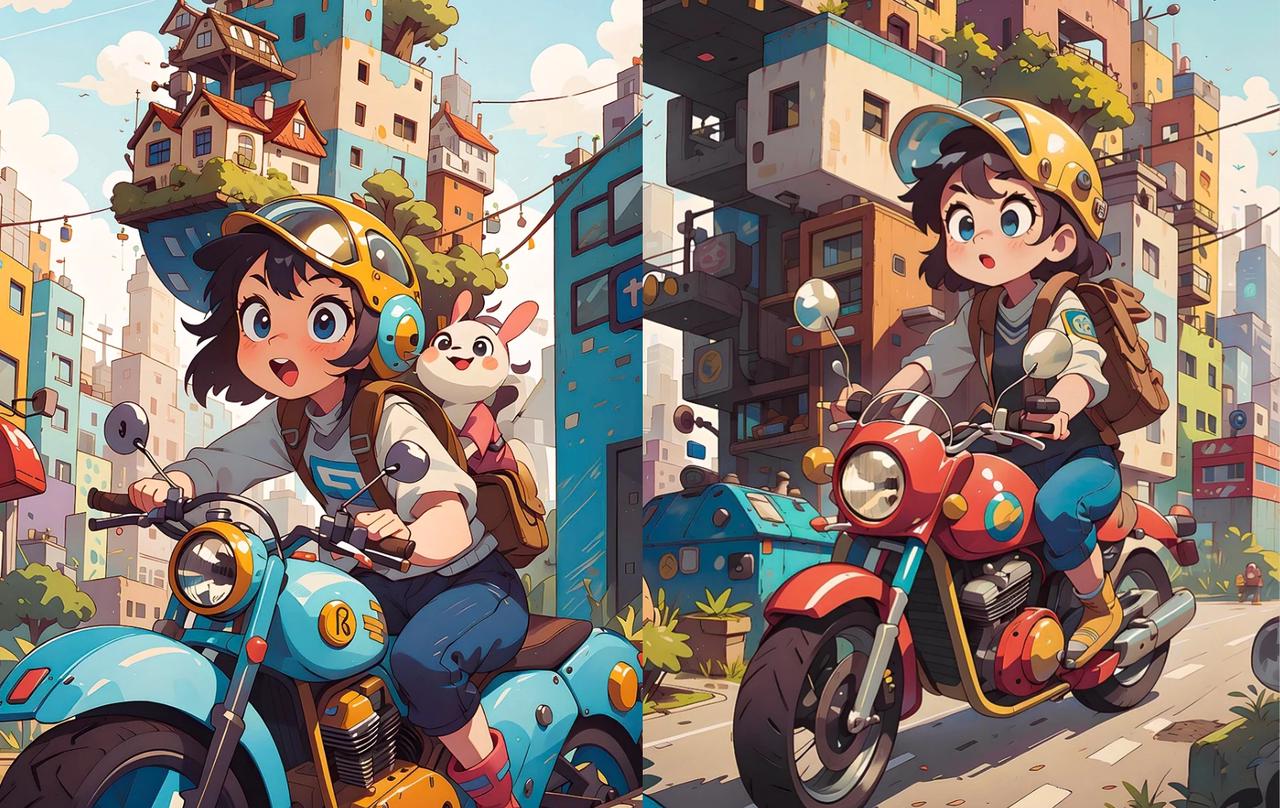
Is VAE Necessary to Install for Stable Diffusion?
The necessity of VAE for Stable Diffusion depends on the specific task at hand. Stable Diffusion is a broad concept that can be applied to various tasks, and not all of them require the use of a VAE. However, in tasks that involve generating new data or transforming data in complex ways, a VAE can be a powerful tool. Therefore, if your Stable Diffusion task involves such operations, installing and using a VAE might be necessary.
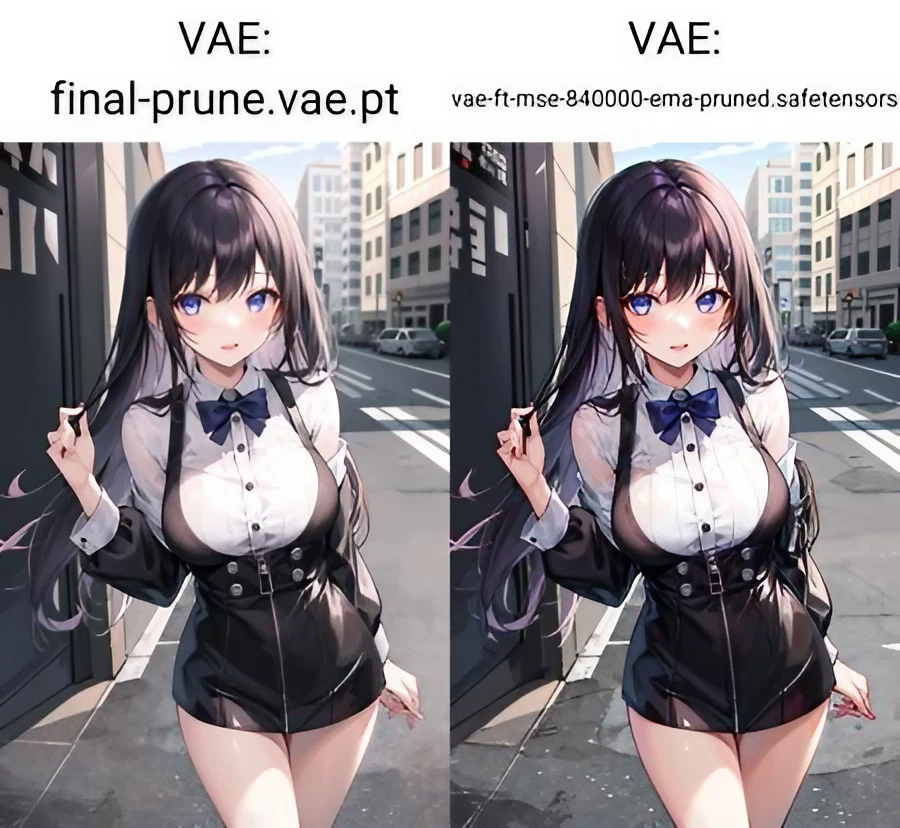
The End!
In conclusion, Variational Autoencoders (VAEs) are a powerful tool in the realm of data science, playing a significant role in certain Stable Diffusion tasks. Understanding when and how to use VAEs can greatly enhance your data science skills and open up new possibilities for your projects. Keep exploring, and happy coding!
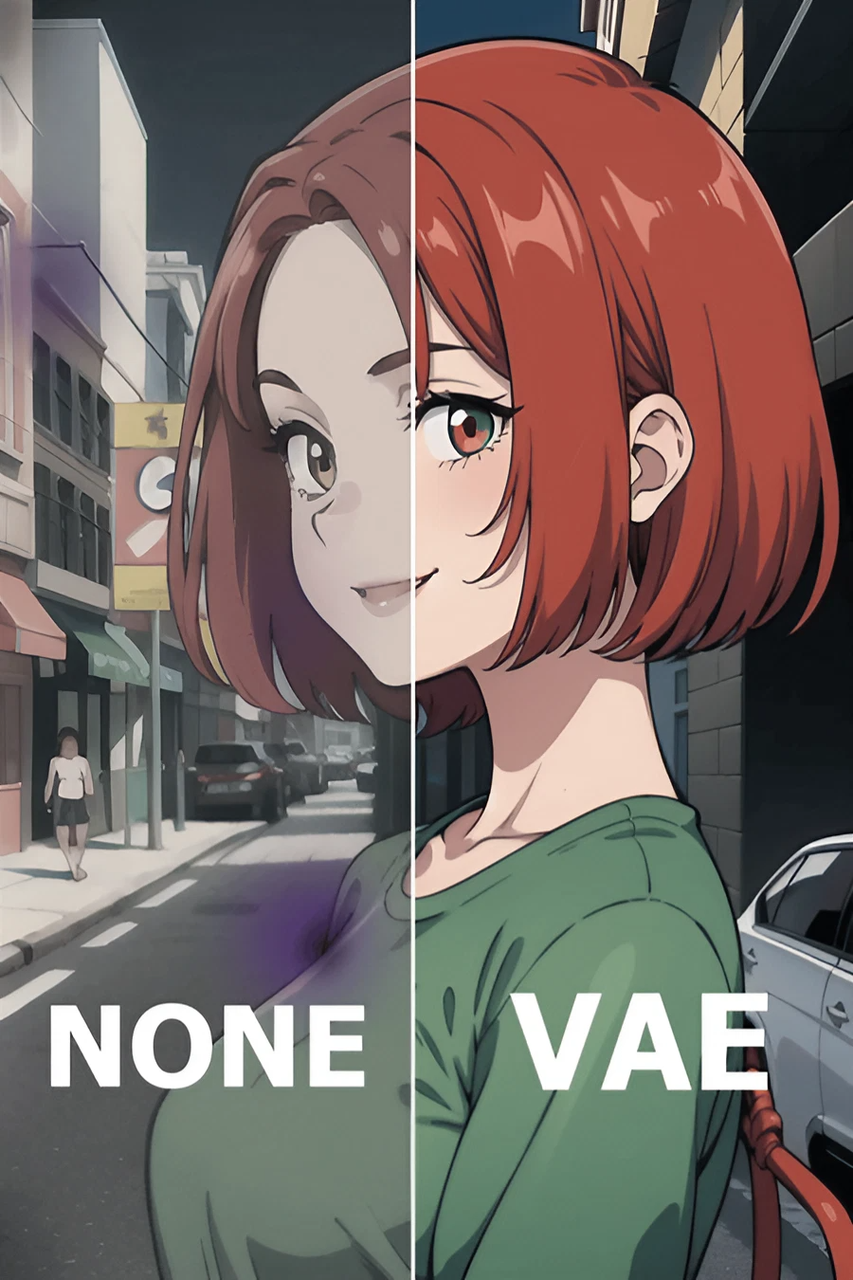
I hope you have a good experience. If you have any other questions, feel free to reach out to me on Discord.
novita.ai provides Stable Diffusion API and hundreds of fast and cheapest AI image generation APIs for 10,000 models.🎯 Fastest generation in just 2s, Pay-As-You-Go, a minimum of $0.0015 for each standard image, you can add your own models and avoid GPU maintenance. Free to share open-source extensions.